Fudan University has intitutional agreements with both the University of Cambridge and the University of Sydney to promote the academic exchange, research collaboration and young scientist training. Despite the disruption of the COVID-19 pandemic which has affected regular physical faculty and student exchanges over the years, we have managed to maintain dynamic activities across various aspects and have strengthened the research links.
This time, we are bringing together three prestigious universities to explore further opportunities for collaboration in the fields of Cognitive Neuroscience, Computational Neuroscience, Neural Engineering and Translational Research.
The aim of this series of symposia organised by and for early career researchers (ECRs) is to foster and promote collaboration as well as people to people exchange. The symposia also serve as a platform for young researchers to present their work and get feedback from their peers. At the same time, it is a hub to find potential international collaborators to start new research projects with.
Ph.D. students and ECRs from the three universities are welcome to sign up as Speaker for future symposia by contacting
istbi_ic@fudan.edu.cn
Instructions for participants
Date:Thursday 15 June 2023
Time:9:00am - 11:00am London, 4:00pm - 6:00pm Shanghai, 6:00pm - 8:00pm Sydney
Zoom ID:849 8739 0705
URL:https://uni-sydney.zoom.us/j/84987390705?from=addon
Registration:https://www.eventbrite.com.au/e/fudan-cambridge-sydney-young-scholar-symposium-2-tickets-639185710667
9:00am - 9:05am London,
4:00pm - 4:05pm Shanghai,
6:00pm - 6:05pm Sydney
9:05am - 9:20am London,
4:05pm - 4:20pm Shanghai,
6:05pm - 6:20pm Sydney
Probabilistic Coding and Learning in Spiking Neural Networks with Correlated Fluctuations
· Yang QI, Postdoctoral Researcher, Institute of Science and Technology for Brain-Inspired Intelligence, Fudan University
9:20am - 9:35am London,
4:20pm - 4:35pm Shanghai,
6:20pm - 6:35pm Sydney
Exploring Neural Heterogeneity in Inattention and Hyperactivity
· Natalia Zdorovtsova, Ph.D. Student, MRC Cognition and Brain Sciences Unit, University of Cambridge
9:35am - 9:50am London,
4:35pm - 4:50pm Shanghai,
6:35pm - 6:50pm Sydney
Deep Learning Applications for Brain MRI Synthesis
· Zihao (Jack) Tang, Ph.D. Student, School of Computer Science & Brain and Mind Centre, University of Sydney
9:50am - 10:05am London,
4:50pm - 5:05pm Shanghai,
6:50pm - 7:05pm Sydney
Small proteins of the global microbiomes
· Yiqian Duan, Ph.D. Student, Institute of Science and Technology for Brain-Inspired Intelligence, Fudan University
10:05am - 10:20am London,
5:05pm - 5:20pm Shanghai,
7:05pm - 7:20pm Sydney
Insights into Emotional Processing from Intracranial Recordings and Their Potential for Biomarker Discovery in Affective Disorders
· Saurabh Sonkusare, Research Associate, Department of Psychiatry, University of Cambridge
10:20am - 10:35am London,
5:20pm - 5:35pm Shanghai,
7:20pm - 7:35pm Sydney
Multimodal Analysis of Neural Dynamics across Neurological Disorders
· Annie Bryant, Ph.D. Student, Department of Physics, University of Sydney
10:35am - 11:00am London,
5:35pm - 6:00pm Shanghai,
7:35pm - 8:00pm Sydney
Speakers Information
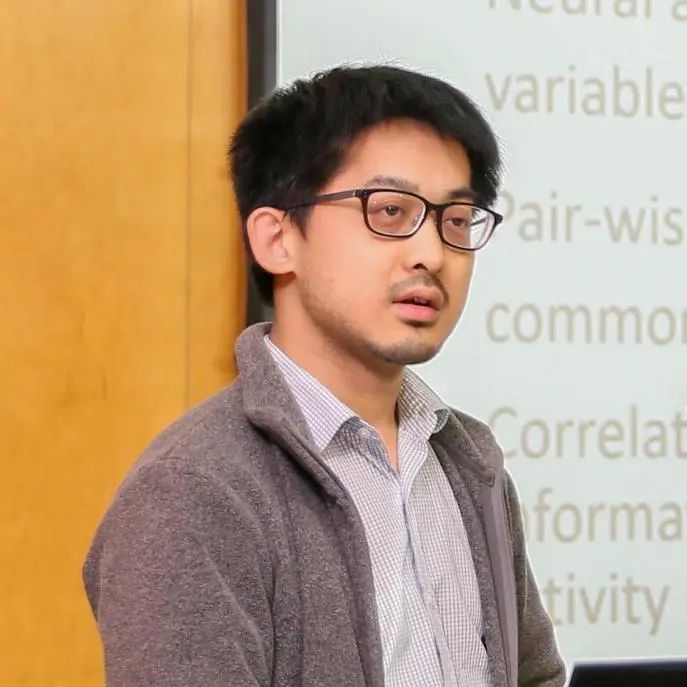
Dr Yang QI
Postdoctoral Researcher, Institute of Science and Technology for Brain-Inspired Intelligence, Fudan University
Biography:Dr. Yang Qi is a postdoctoral researcher in the Institute of Science and Technology for Brain-inspired Intelligence (ISTBI), Fudan University, China. He obtained his doctorate degree in Physics from the University of Sydney, Australia. His main field of research is computational neuroscience with interests in spatiotemporal dynamics of neural circuits, probabilistic neural computation, neural coding theories, and machine learning. His recent research involves developing learning algorithms for spiking neural networks and stochastic neural computing theories.
Presentation Title:Probabilistic Coding and Learning in Spiking Neural Networks with Correlated Fluctuations
Abstract:Inspired by the highly irregular spiking activity of cortical neurons, stochastic neural computing is an attractive theory for explaining the operating principles of the brain and the ability to represent uncertainty by intelligent agents. However, computing and learning with high-dimensional joint probability distributions of spiking neural activity across large populations of neurons present as a major challenge.
To overcome this, we develop a novel moment embedding approach to enable gradient-based learning in spiking neural networks accounting for the propagation of correlated neural variability. We show under the supervised learning setting a spiking neural network trained this way is able to learn the task while simultaneously minimizing uncertainty, and further demonstrate its application to neuromorphic hardware. Built on the principle of spike-based stochastic neural computing, the proposed method opens up new opportunities for developing machine intelligence capable of computing uncertainty and for designing unconventional computing architectures.
Natalia Zdorovtsova
Ph.D. Student, MRC Cognition and Brain Sciences Unit, University of Cambridge
Biography:The goal of my research is to uncover how networks in the brain develop change childhood and adolescence, and how this process of development might differ in people with particularly high levels of inattention and hyperactivity. I use MRI, fMRI, and MEG to understand the brain, and my methodology is a transdiagnostic one.
Presentation Title:Exploring Neural Heterogeneity in Inattention and Hyperactivity
Abstract:Inattention and hyperactivity are cardinal features of Attention Deficit Hyperactivity Disorder (ADHD). However, these characteristics are not exclusive to ADHD—they have been observed across a range of other neurodevelopmental conditions, such as autism and dyspraxia, which suggests that they might best be studied across diagnostic categories. In this presentation, I’ll be discussing our recently-published study (doi: https://doi.org/10.1016/j.cortex.2023.04.001), in which we evaluated the associations between inattention and hyperactivity behaviours and features of the structural brain network (connectome) in a large transdiagnostic sample of children (Centre for Attention, Learning, and Memory; n = 383). In our sample, we found that a single latent factor explains a high degree of variance in participants’ scores across multiple questionnaires measuring inattention and hyperactivity, but Partial Least Squares (PLS) regression indicated that variability in this latent factor could not be explained by a linear neural component.
We then investigated the type and extent of neural heterogeneity in a subset of our sample with clinically-elevated levels of inattention and hyperactivity. Multidimensional scaling combined with k-means clustering revealed two neural subtypes in children with elevated levels of inattention and hyperactivity (n = 232), differentiated primarily by nodal communicability—a measure which demarcates the extent to which neural signals propagate through specific brain regions. These different clusters had similar behavioural profiles, which included high levels of inattention and hyperactivity. However, one of the clusters scored higher on multiple cognitive assessment measures of executive function. We conclude that inattention and hyperactivity occur across different neurodevelopmental conditions because they’re capable of emerging through multiple different trajectories of brain development. In our own data, we can identify two of these possible trajectories, which are reflected by measures of structural brain network topology and cognition.
Zihao (Jack) Tang
Ph.D. Student, School of Computer Science & Brain and Mind Centre, University of Sydney
Biography:Zihao Tang is a Ph.D. candidate at the School of Computer Science and the Brain and Mind Centre. His research topic focuses on deep learning applications for brain MRI synthesis. He has completed several projects on synthesis for both structural and diffusion MRIs during his Ph.D. candidature. He is dedicated to proving the feasibility of utilizing deep learning applications in MRI-related tasks from a cross-disciplinary perspective.
Presentation Title:Deep Learning Applications for Brain MRI Synthesis
Abstract:Deep learning methods have been applied to various MRI related tasks, including segmentation, classification, and registration, due to their impressive performance. However, the application of MRI synthesis poses a dilemma due to the absence of gold standard in most scenarios. In this presentation, I will show several studies we have conducted on this topic and demonstrate how we prove the feasibility of using deep-learning-based method on MRI synthesis tasks from not only the computer science but also the clinical perspectives.
Yiqian Duan
Ph.D. Student, Institute of Science and Technology for Brain-Inspired Intelligence, Fudan University
Biography:Yiqian Duan is a Ph.D. student in the Institute of Science and Technology for Brain-inspired Intelligence (ISTBI), Fudan University, China. Her main research focus is large-scale metagenomics analysis and especially regarding global host-associated microbiomes. She recently applied bioinformatics methods to study microbial small proteins in the global microbiomes.
Presentation Title:Small proteins of the global microbiomes
Abstract:Small proteins have important biological functions, and they are usually excreted from the cells that produce them. If they are microorganisms in the human gut, small proteins and peptides can enter the bloodstream and may trigger immune responses such as inflammation. However, small proteins are often ignored in experimental research and computational methods due to their small size. Hence, we built a comprehensive global microbial small proteins catalogue containing taxonomy and habitat annotation. Based on this catalogue, we have uncovered their basic ecological distribution patterns. And some microbial small proteins with structural characteristics can perform crucial roles associate to human health. For example, antimicrobial peptides have been analysed in a variety of medical applications, which can be used to treat cancer or fight against a variety of antibiotic resistant bacteria to solve the problem of drug resistance. By cataloging the functional diversity of small proteins from around the world, we hope to provide new insights into how these molecules modularize interactions between microorganisms and hosts (such as humans and human gut microbiome).
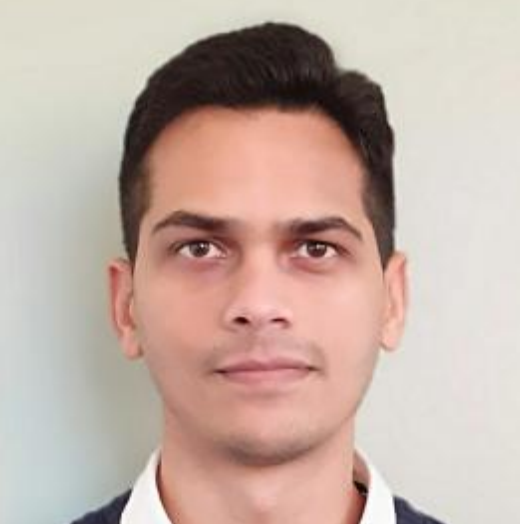
Saurabh Sonkusare
Research Associate, Department of Psychiatry, University of Cambridge
Biography:I have primarily been trained and worked as a medical doctor (MBBS) with a subsequent PhD in Neurosciences from QIMR Berghofer/The University of Queensland, Brisbane, Australia. I utilise neuroimaging (LFPs, fMRI) and neuromodulation tools (TMS) to understand the functioning of key brain regions, their interactions, and meso/macroscale brain dynamics. I also specialise in psychophysiological measures (heart rate and skin conductance) and especially facial thermal imaging having developed methods for its analyses. Using traditional task paradigms as well as naturalistic ones such as movies, overall, these streams of research form the basis for my investigations on emotions, interoception, their neurobiological underpinnings, as well as their breakdown in affective disorders and anxiety disorders including OCD.
Presentation Title:Insights into Emotional Processing from Intracranial Recordings and Their Potential for Biomarker Discovery in Affective Disorders
Abstract:Intracranial recordings provide the distinct advantage of direct high fidelity signals at neuronal population level providing the means to uncover the precise frequency dependent activity and coupling among brain regions. Such knowledge is of significant value in the evolving field of neuromodulation (at node/network level) to understand the neurobiological basis of emotional processing and its dysfunction. In this talk I will first show how intracranial recordings from the amygdala, orbitofrontal cortex and medial prefrontal cortex allow investigation of emotional valence differentiation and the connectivity profile. Furthermore, to highlight the translational potential of such intracranial recordings, I will present results from a study of human habenular recordings investigating valence differentiation and non-oscillatory component of the power spectrum which can be used to characterise the severity of depression.
Annie Bryant
Ph.D. Student, Department of Physics, University of Sydney
Biography:I’m a second-year PhD student in the Dynamics and Neural Systems lab led by Dr Ben Fulcher at The University of Sydney. Here, I think about ways to measure differences in neural activity dynamics in diverse neurological disorders and how to translate such differences into novel potential avenues for treatments. Before joining this lab last year, I worked as a research technician in an Alzheimer’s disease lab at Massachusetts General Hospital/Harvard Medical School, leveraging multimodal -omics and neuroimaging datasets to study the intersection between tau pathology and vascular function.
Presentation Title:Multimodal Analysis of Neural Dynamics across Neurological Disorders
Abstract:Functional neuroimaging techniques like fMRI and EEG present a non-invasive window into neural activity dynamics in the human brain, which is relevant to both basic science and clinical applications. We propose a highly comparative approach to quantifying patterns in neuroimaging time series that integrates both univariate (local, regional dynamics) and pairwise (region-region coupling) structure. This allows us to comprehensively characterize underlying differences in neural activity across domains – from schizophrenia to mild cognitive impairment. I will share some of my thesis results demonstrating the utility of this framework in a neuropsychological classification setting, along with my proposed next directions extending this to Alzheimer’s disease neuropathology.
For collaborative research, academic visits, organization of academic events and other international activities with us, please contact istbi_ic@fudan.edu.cn